.png)
You’ve got hundreds of resumes to wade through for a position you need to fill quickly. You’re wary of using human screeners because you know they could make snap judgements and accidentally reject highly qualified candidates.
You don’t want to cut corners on background screening, but you need to hire someone and start onboarding ASAP. So, why not use an AI-based candidate screening tool to get the job done quickly? After all, a machine prevents bias rather than amplifies it, right?
Sadly, this isn’t the case. AI recruitment technology can exaggerate the same biases found in human recruiters. And the problem is so widespread that New York City recently passed Local Law 144 that prevents employers from using AI recruitment software unless they publish:
- a declaration on the company website
- the distribution date of the tool
- a summary of the tools’ most recent bias audit.
Penalties for flouting the new legislation (introduced from 15th April, 2023) range from $500 to $1,500 per offence.
Our guide examines why AI-based screening tools may be biased and offers a fairer type of candidate screening software to introduce into your hiring process.
5 ways your candidate software could be biased
AI-powered screening software may shave time off your hiring process by reducing the amount of steps and repetitive tasks involved. But it can be riddled with bias at multiple points causing you to hire sub-par candidates just because they got straight As at school or previously worked for a big-name employer.
Here are five ways your applicant screening tools may stop you reaching your recruitment targets.
1. Job ad presentation
AI recruiting relies on algorithms designed to present the most relevant information (in this case, job ads) to a specific audience. This delivery of job board postings reinforces gender and racial stereotypes as candidates can only apply for your role if they see your job listing in the first place. And if you’re not reaching a diverse audience, that means your talent pool could end up looking very similar.
Example: a study of Facebook ads revealed that ads for supermarket cashier positions were presented to an audience of 85% women, while job ads for taxi companies were visible to a 75% Black audience.
This happens because some advertising platforms use a recommendation system to replicate trends in user behaviour and preferences. Meaning that some potential jobseekers will be more likely to see your job ad than others. For example, if recruiters regularly interact with white males, the software will present job ads to a similar demographic. Artificial intelligence algorithms do this by finding correlations between names, neighbourhoods, and hobbies, then replicating this pattern.
2. Resume screening
CVs are an open door for bias to sneak into the recruitment process, which is why Applied doesn't recommend using them. But if they're still an integral part of your hiring funnel, AI-powered screening tools are a tempting way to save time sifting through thousands of resumes.
Resume screening software uses algorithms to scan resumes and cover letters for information related to job requirements, such as experience and qualifications. But these tools can also prioritise resumes based on candidates’ protected characteristics such as:
- Gender
- Age
- Education level
- Race
The algorithms are often designed to select candidates which are similar to the employer's past successful hires. So, they might inadvertently favour candidates using .edu email addresses as an indicator of age, or specific postcodes which might be linked with an affluent neighbourhood. These micro-biases add up, meaning your candidate crop may be much more homogenous than you think, leading to the same kind of discrimination that is seen in manual recruiting processes.
A prime example of a biased resume screening model was Amazon’s, which was abandoned after it showed a strong preference for male candidates. The algorithm learned to discount CVs containing the word “women’s”, and penalised those that had attended all-female college courses.
Of course, no one deliberately built this machine to be sexist. But they did intend it to observe hiring trends based on resumes submitted over a decade. The problem? As the majority of hires were men during this time, the machine learning system could only assume Amazon preferred male over female candidates.
3. Skills keywords
Recruiters are turning their attention to focusing on skills rather than candidate experience or education during the hiring funnel. This approach can accurately predict whether a candidate has what it takes to succeed in the role.
So, it’s understandable that recruiters use screening tools that rely on resume keywords as a way to identify candidates that match with the job description.
But research shows that women are more likely to downplay their skills, while men are prone to exaggerating their skills and experience. The result? AI can only work with the keywords available, so if there's an imbalance in language between the genders, or an overrepresentation of a particular type of candidate, the system will skew towards their resumes.
4. Gamification tests
Gamification is gaining pace in recruitment, with employers using gaming-style assessments to delve into candidates' personalities, cognitive skills and aptitude for the role.
These tests are based on data gathered from years of previous candidates and their performance. But if the data favours one type of candidate, it's likely that the algorithm behind the gamified test will be biased too.
Additionally, gamification can cause disability discrimination facilitated by algorithms, which is a growing problem acknowledged by the Equal Employment Opportunity Commission (EEOC). Specifically, this type of hiring can unintentionally screen candidates straight into the rejection pile if the platform isn't accessible enough or they're unable to navigate the game because recruiters haven't made accommodations for their disability.
5. One-way video interview screening
Asynchronous video interviews are a tactic that have clear scheduling advantages for both recruiters and candidates. Jobseekers record a video of themselves answering questions before recruiters review their responses in their own time. This allows hiring panels to process job applications faster and share recordings with stakeholders involved in the recruitment process.
Some video screening software uses speech recognition technology to interpret answers, but research shows that this type of AI can be biased against certain accents and genders. Additionally, Carnegie Mellon University found that speech recognition tech doesn’t understand atypical speech well, such as the speech of deaf people.
Similarly, some 700,000 UK people are on the autism spectrum and this condition can also impact speech patterns. As such, these technologies may unintentionally exclude some very qualified individuals with disabilities or those who aren’t neurotypical from the application process.
How to reduce bias from your AI screening tool
If you’re hoping to simplify your hiring process using AI but don’t want to invite bias, there are several steps you can take to ensure you’re attracting and hiring the most qualified active and passive candidates.
Spoiler alert: you won’t necessarily be recruiting more of the same (and that’s a good thing.)
Step 1
Do your due diligence before integrating a tool into your hiring process. Liaise with software vendors to confirm that you’ll have access to candidate selection data, to understand which candidates you’re turning away and why.
Step 2
Once you’ve committed to a tool, set up a regular audit cycle to examine the results of your accepted and rejected candidates. If you determine a negative link between application rejection and protected classes, it’s imperative you take action to prevent future discrimination. The best practice here would be to keep in touch with rejected candidates and inform them about alternative roles they might be a match for.
Step 3
Ensure you use a diverse set of input data to inform your algorithm. The more diverse the data, the less likely your algorithm will be to form automated generalisations and perpetuate stereotypes. Conversely, if you've only ever hired white men aged 25 to 45, then you can expect your algorithm to reject candidates outside of this demographic.
Replace AI screening with a fair and predictive alternative
AI will only be as good as the data that feeds the algorithm. And as we’ve seen, there are multiple examples of machine learning bias that can creep into recruitment processes.
If you’re looking for technology that simplifies your recruitment workflows, while improving your quality of hire, let us introduce you to Applied.
Our applicant tracking system identifies the ideal candidate for your role and forecasts how well they’ll perform once they’re up and running. The technology uses a human approach to the applicant screening process, but incorporates bias-elimination techniques like the following to ensure a fair outcome:
Anonymised screening
Blind hiring is the process of removing identifiers, such as names, ages, genders, addresses, photos, or anything else from a candidate’s CV that could influence the hiring decision. Instead, our ATS serves up data identifying candidate skills that are relevant to the role they’re applying for.

Work samples
What better way to predict how a job applicant will fare in a role than by asking them to submit a sample of work that relates to the everyday tasks they’ll be asked to perform? You can also ask them to complete a small exercise and discuss their approach to the challenge. Here’s an example of a work sample question:
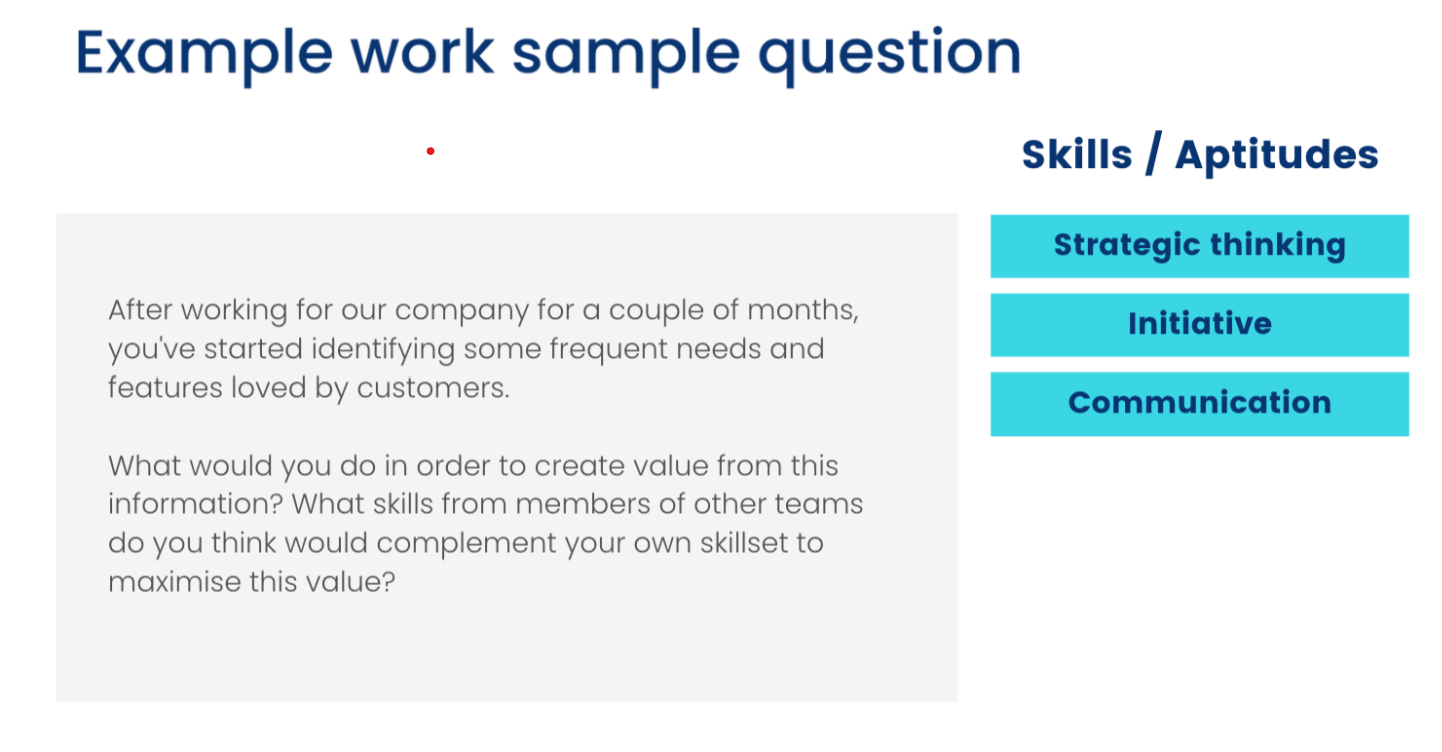
Skills-based shortlisting
Once you have a pool of talented potential candidates to choose from, the next step is to shortlist those with the specific set of technical skills you require. Applied uses chunking to break individual applications into pieces and groups answers to the same questions together. The candidate answers are also randomised so recruiters aren't influenced by ordering effects.
.png)
Cognitive testing
Our tool includes several tests to measure information processing and knowledge retention. Assess cognitive ability using the following tests:
- Maths skills
- Data interpretation
- Analytical thinking
- Error spotting
.png)
Applied is a purpose-built recruitment automation software that fairly and accurately screens candidates based on their skills. Choose from a wide range of cognitive, numerical, personality assessments, and structured interviews to reliably predict which candidates will excel at your organisation. Book in a free demo today.