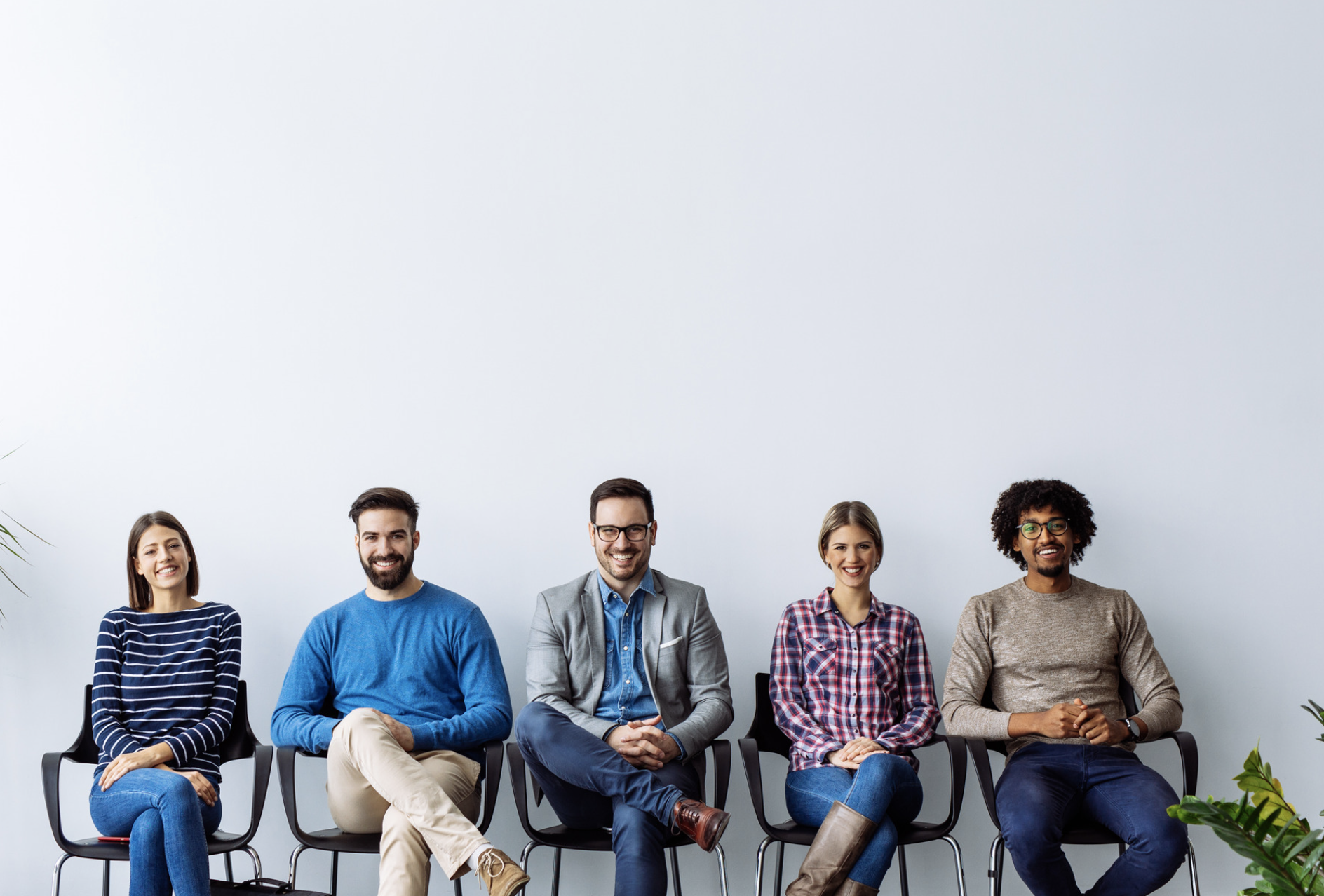
Originally posted on Medium, 26 April, 2018
Inspired to share by a great post by Jean Hsu and Jeff Lu, here I’ll outline how we decided what kind of hiring pipeline to build, and the 3 assessment techniques we chose to build out in our product.
“From the point of view of practical value, the most important property of a personnel assessment method is predictive validity: the ability to predict future job performance” — Schmidt/Hunter (1998)
Hiring like a cave-bro
I’ve always sucked at hiring. I want people to like me, so I pick CVs like mine, and spend the typical interview making friends, and forget that my job is to assess their ability as objectively as I can. It becomes about rapport… how much people fit in socially.

Those aren’t my only failings as a hiring manager, either.
- I skim read peoples CVs so miss important details
- I mentally over-value skills that reflect well on me, and under-value skills that I don’t possess
- I make snap judgements at interview, so some candidates start at an advantage
- I don’t know what to ask candidates, which questions reveal their ability the best
- I ask different candidates different questions, so each interview is different and hard to compare to others.
- When discussing candidates with other interviewers I too often agree when I actually disagree
Pretty bad, right?
Maybe one or two sound familiar, the evidence seems to show that being bad at this stuff is pretty standard (although I’m sure the details and severity vary).
Why not just… do better?
Some of this stuff is easily fixed. For example, I could be more structured with my interviews to make them more consistent… but there are things here that are hard to fix through force of will alone, such as letting first impressions unduly influence my opinions.
Ultimately I want to help everyone else solve this stuff too, which means changing the behaviour of people I’ve never met.
Behaviour change is hard, and changing the behaviour of entire companies is even harder, let alone beyond that.
How about bias training?
There’s very little evidence that training works, in fact in some cases it can be counter-productive. It’s also hugely expensive and time consuming.
Behaviour change is hard, and changing the behaviour of entire companies is even harder, let alone beyond that.
How about pushing for legislation?
The job of the law (in my opinion) is to define the desired outcome and provide an avenue for justice if things go wrong. While there may be ways it could be improved, we’re broadly already there. The problem on the other hand is in the detail of process design, and legislation is too slow and blunt a hammer to unscrew that mixed metaphor. We wanted to empower HR and hiring managers, not bog them down in the labour required to run a de-biased hiring process.
So…
We decided to build tools
If we can’t rely on training, or regulation, that leaves tools. It means building hiring tools that we use ourselves, and convince other people to use.
But there are so many options, how do we know what to build?
(Note for later: In the straight-to-video screenplay of my life we should do a montage here, mostly of Kate reading basically all of the science while I say stupid shit on Twitter.)
Enter Schmidt and Hunter, in slow motion… to AC/DC
Back in 1998 (when the Spice Girls were huge, Justin Timberlake was the dorky one in N-Sync, and Madonna’s Ray of Light album finally clued the US into the fact that dance music is a thing, which they then preceded to rename “EDM” and get entirely wrong) Frank Schmidt and John Hunter published a meta-study pulling together 85 years of research on how to assess candidates.
It’s a nutrition label for hiring processes
The key output of their study is a simple table (below) showing predictive validity of assessment types, i.e. how likely it is that someone who does well at each type would actually turn out to be good at the job.
(This paper may as well have been delivered to the publisher while walking down the road, in sunglasses, with “Back In Black” playing in the background).
The top validity score here, r=0.54, is actually pretty mediocre, you wouldn’t want to hire on that alone, so you can see why hiring methodology has built up layers of assessment to improve the odds of making a good hire. The data fits.
But to achieve a low false positive rate (bad hires) using layered assessments you need to discard people who fail any one stage… so it follows that low-validity assessments typically also have a high false negative rate (good people turned away), meaning you need to process far more candidates to get the same number of hires.
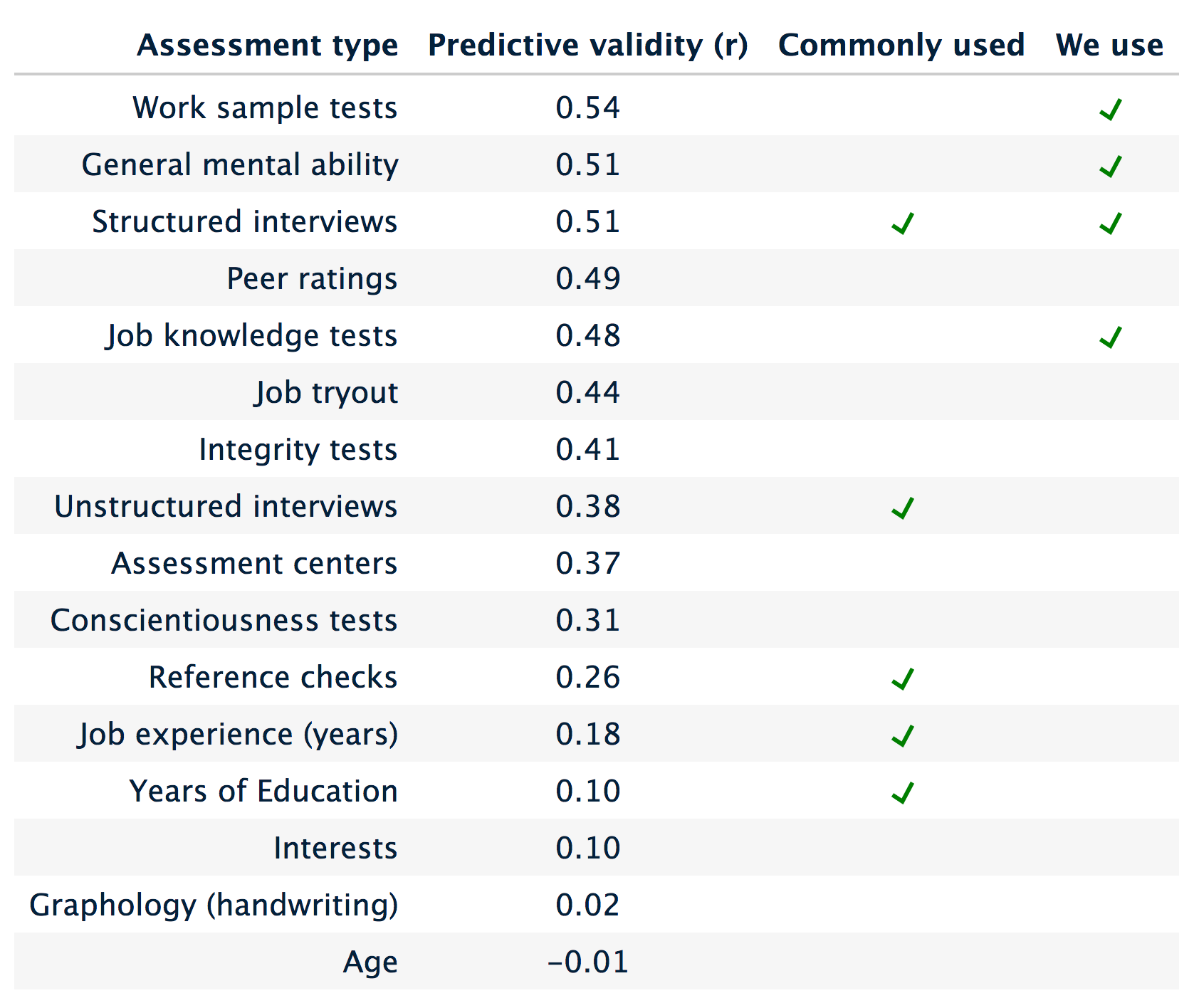
But what’s most striking about this table is that the assessments most companies use have very low predictive validity. Most companies don’t even use structured interviews, so they best they’re getting is a cross-reference between a handful of very weak indicators.
CVs, for example, contain some of the weakest indicators of ability and the strongest sources of potential bias… peoples names, age, the names of institutions they studied at, their years of experience in an area, and the names of companies they worked at.
(Quick note: we ran a study to test this and our tools had triple the predictive power of even a well-run CV process that had no gender or ethnic bias… fist bump... mic drop).
Not only do individuals broadly suck at hiring, but companies suck at designing hiring processes.
How we decided what to build
As you‘ve guessed we thought it’d be worth trying something different.
We’re looking at a complex problem, but we know the boundaries of it, and thanks to Schmidt and Hunter, as well as all the people researching different aspects of hiring bias, we have rough hint of a recipe.
Our approach
- Take the most predictive types of assessment
- Look at the ways those assessments could work at scale, what known bias problems they’re susceptible to, and how easily we can supply the content and supporting tech
- Build tools that support those processes while minimising any bias risks
- Get real data from real hiring rounds
- Continuously monitor each part of the process for both bias and predictive power*
*Note: One day we might have to make a trade-off between predicting the best candidate and supporting diversity, but we haven’t yet been faced with that. So far (touch wood) the two things seem very closely aligned.
Our winning assessments
It’s about time I got to the point and told you what we’ve tried out so far. So far on our platform we’ve implemented (and used both internally and with customers):
1. Work samples
These are just written answers to questions directly relating to the job. They typically test some combination of job knowledge and situational judgement, and are most effective when covering types of work that accurately reflect the job itself.
These answers are rated by a human review team in the hiring organisation, in such a way as to reduce affinity bias, stereotype bias, halo effects, confirmation bias, ordering effects, comparison friction and groupthink… but that’s a story for another blog post.
2. Structured interviews
To make it harder to base interview results off first impressions, biases, or lack of discipline, we built out a process through which hiring managers can define the structure and content of interviews, and interviewers can record their notes and assign scores. The gains here come from ensuring consistency across candidates, helping teams focus on the skills important to the role, and pushing interviewers away from ‘System 1’ thinking towards ‘System 2’ thinking (and hence away from all the associated cognitive biases).
3. Multiple-choice tests (yep)
Multiple choice tests (while obviously limited) are a great tool for larger scale rounds where time constraints demand a filter that scales with candidate volume. They can be used either to test basic domain knowledge, or for general skills, e.g. numeracy.
We see these tests as having the loosest correlation with performance of the three, mainly due to the limiting format of multiple choice. We’d recommend using these as a loose filter, i.e. use the resulting scores to drop the bottom 20%, not the bottom 80%, but broadly speaking it’s a great way to manage bulk hiring. We tend not to use these for smaller rounds or more senior hires.
The commercial numeracy testing market also had severe problems with gender and minority bias to the tune of 8% to 25%, (the gender skew in maths at key stage 4 is around 3%). To illustrate how bad that skew is in practice; if you set a pass mark at the 80th percentile with the industry leading numeracy test provider, you’d get three times more men than women. That’s 3x. Triple. 300%. Our numeracy test still has a skew, but it’s the lowest in the sector and we’ve only just started.
That’s all for now
We’ll follow up with some posts later showing some data analysis of these talent assessment types. Here’s a sneak peak of some work in progress to show how we go about unpicking which interview questions are fair and objective, and actually predict job performance.
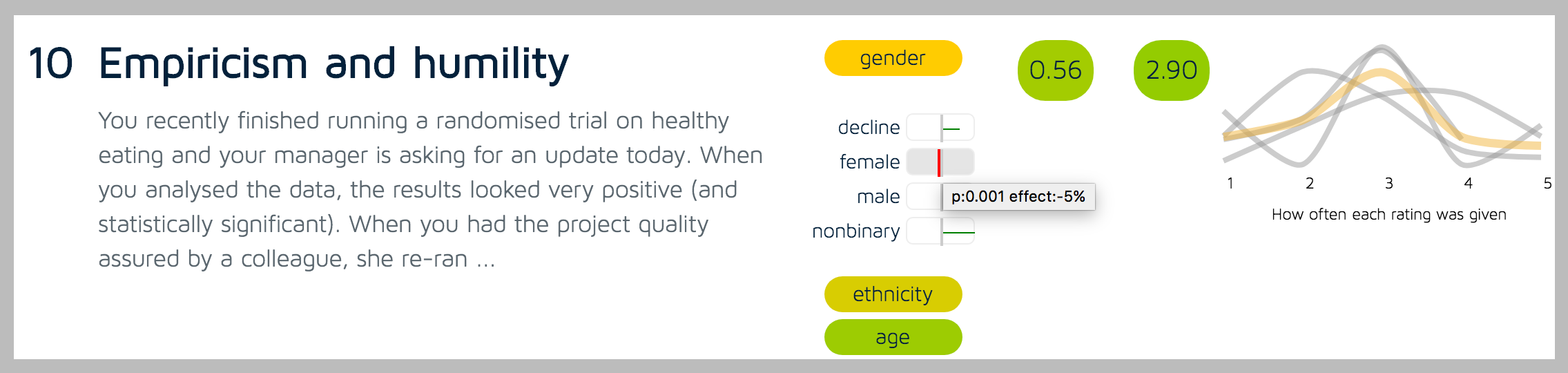
In the meantime we’d love to hear what you’re using, and where you think we could be doing things better.